DLEAM - Deep Learning Additive Manufacturing
DLEAM
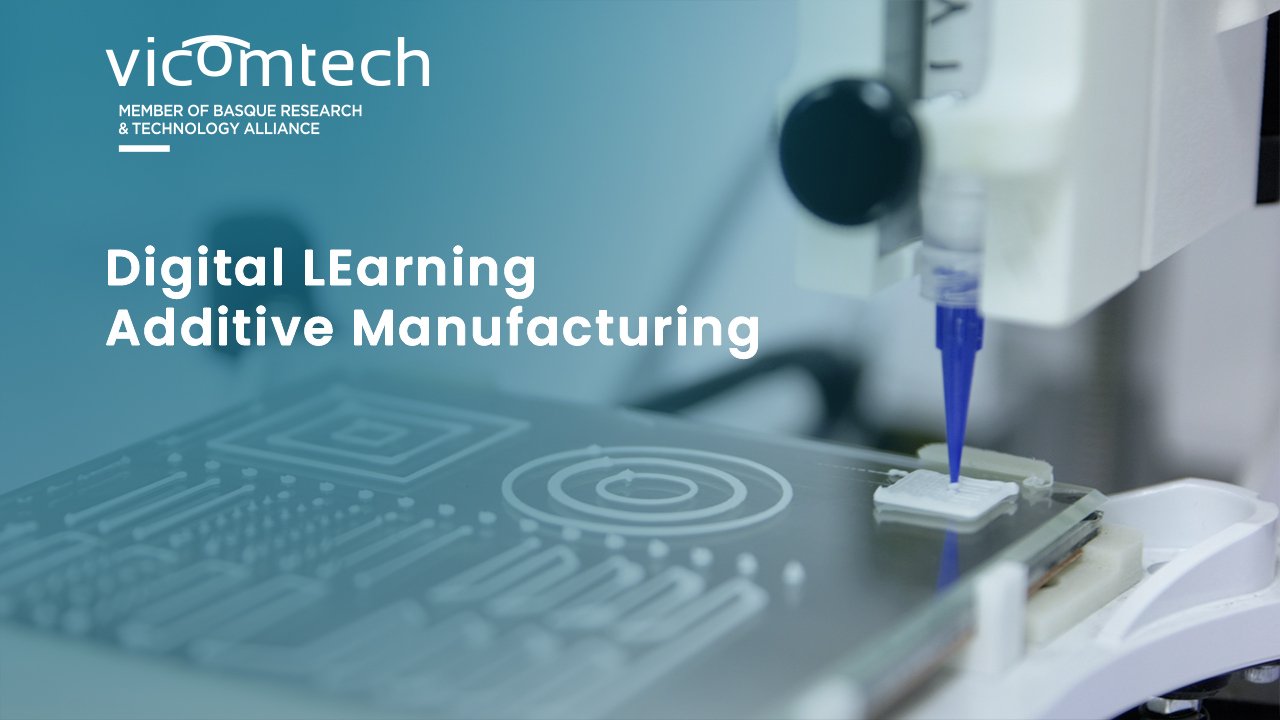
Context
Additive Manufacturing has evolved rapidly from “Rapid Prototyping” to “production-ready” parts. This technology is critically relevant in many areas, by supporting the fabrication of aeronautic, metallurgic, plastic and biologic parts. It benefits manufacturers in the design and modelling of innovative structures, end-product readiness, and time-to-market. The implications of this technology are vast and could revolutionize our society. For instance, production could take a turn into small enterprises, reinforcing the local economy. Medicine could be revolutionized by low-cost prosthetics and personalized printed organs and pills. This would increase the efficiency of pills, make prosthetics available in war-torn zones and reduce transplant problems. Recycled metals, plastics or papers could be the ink for printers, helping to save the environment.
Technological Challenges
This project aims to design and develop innovative and disruptive methodologies that facilitate the characterization of the printing tool parameters when a new printing material or machine is introduced in the process.
Challenges:
-
Machine vs. Material Calibration
-
Human Factor: Trial & Error
-
Waste of resources
-
Non-standarised protocols
-
Process variability/uncertainty
Methodology
The overall methodology has been the calibration of Machine parameters based on Material/Geometry characterization
Modules:
- Machine Learning based Model
- Computer Vision Techniques
- CAD, CAM, CAE
- CFD (Computer Fluid Simulation)
- Rheology Study (In collaboration with UPV/EHU & BioMat)
- DoE (Design of Experiment) & R&R
Technical Results
The modular design of the project enabled the generation of “independent” results between phases or modules
Main:
- ML – Prototype for Parameter Estimation
Side:
- Bioprinting CALIB dataset
- In-situ Computer Vision Systems
- Bioprinting Protocol/Standardization
Scientific Results
In-process:
- A Machine Learning Model for Machine Parameter Optimization
- Towards Bioprinting Protocol Standarization. An R&R approach
- Bioprint-CALIB Dataset.
- Computer Vision System for precise nozzle height calibration
- A Computer Vision / Computational Geometry library for geometric characterization.
- Bioprinting parameter optimization using CFD & Rheology analysis
Applications
- Automation of manufacturing
- Biomaterial Design
- Drug testing
Looking for support for your next project? Contact us, we are looking forward to helping you.